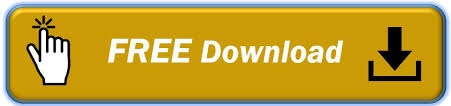
Normally you'll be guided to things in the movies, the screen won't be cluttered too much, there won't be many things to take your eyes away from the main clue or action.
#Trakaxpc text bar movie
The comedy and the emotion is conveyed through the subtlest of movements and it works superbly well.Watching the movie you have to be aware of the entire screen. Park manages to give us everything we need from this silent character through his expression. He doesn't speak, he doesn't make any noise, all he has are his eyes, brow, and body posture, and with these he commands the film. The first is subtlety and observation, the flagship of which is the character of Gromit. Step 2000: eval WeightedCategoryAccuracy | 0.84062500Īfter training the model, run it like any layer to get results.Įxample input_str: There are a few aspects to Park's movies, and in particular Wallace & Gromit, that I would say make them so great. Step 2000: eval WeightedCategor圜rossEntropy | 0.37591279 Step 2000: train WeightedCategor圜rossEntropy | 0.29129386 Step 2000: Ran 500 train steps in 8.54 secs Step 1500: eval WeightedCategoryAccuracy | 0.85117188 Step 1500: eval WeightedCategor圜rossEntropy | 0.35194683 Step 1500: train WeightedCategor圜rossEntropy | 0.35241464 Step 1500: Ran 500 train steps in 9.13 secs Step 1000: eval WeightedCategoryAccuracy | 0.79531250 Step 1000: eval WeightedCategor圜rossEntropy | 0.41775789 Step 1000: train WeightedCategor圜rossEntropy | 0.35916388 Step 1000: Ran 500 train steps in 8.89 secs Step 500: eval WeightedCategoryAccuracy | 0.81406250
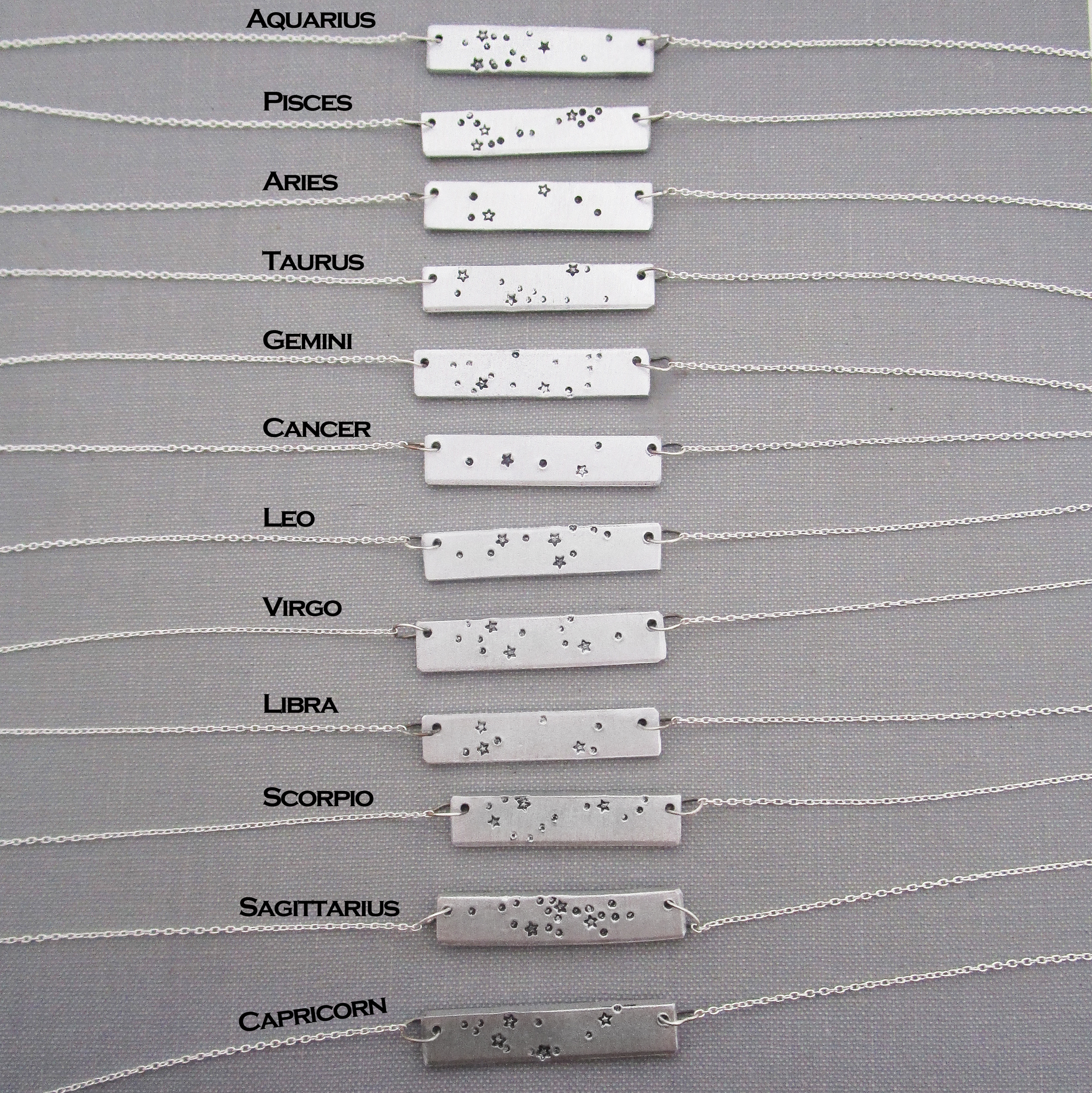
Step 500: eval WeightedCategor圜rossEntropy | 0.42969493 Step 500: train WeightedCategor圜rossEntropy | 0.50712883 Step 500: Ran 499 train steps in 10.62 secs
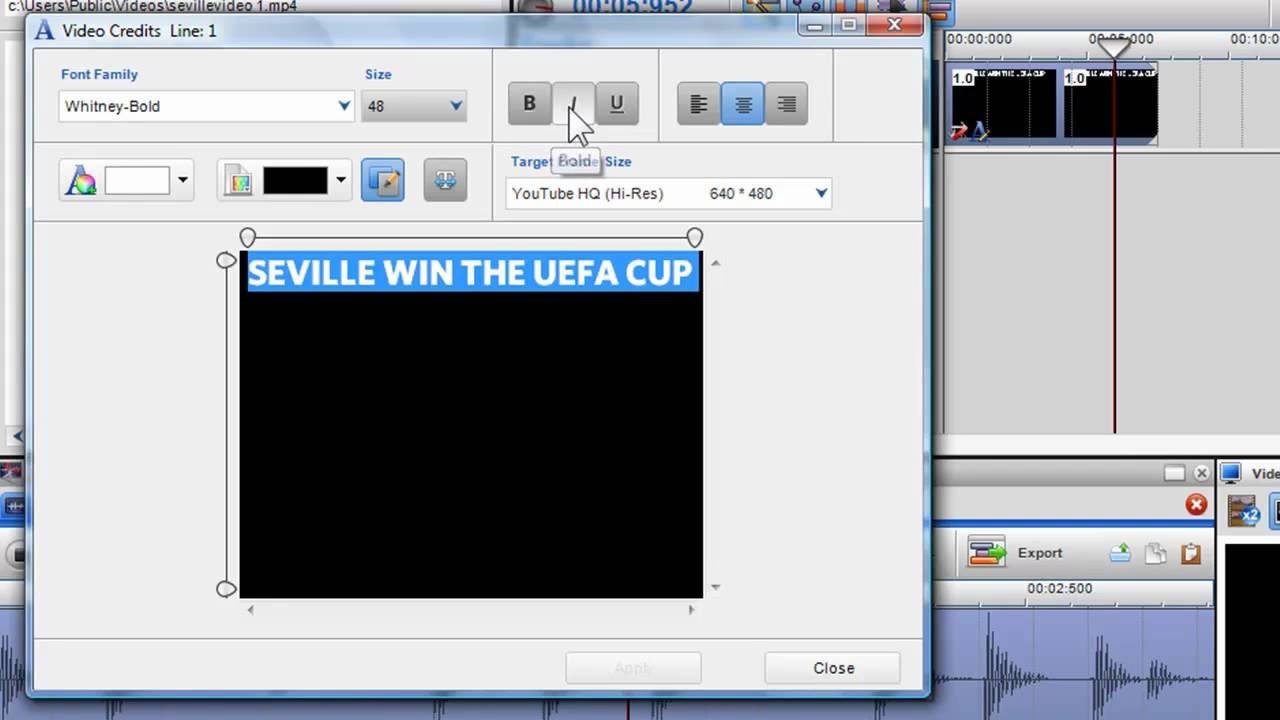
Step 1: eval WeightedCategoryAccuracy | 0.50312500 Step 1: eval WeightedCategor圜rossEntropy | 0.69349981 Step 1: train WeightedCategor圜rossEntropy | 0.69192106 Step 1: Total number of trainable weights: 2097666 Layers with trainable weights like Embedding need to be initialized with the signature (shape and dtype) of the input, and then can be run by calling them. W = self._kernel_initializer(shape_w, self.rng) Shape_w = (self._vocab_size, self._d_feature) """Randomly initializes this layer's weights.""" Return jnp.take(self.weights, x, axis=0, mode='clip')ĭef init_weights_and_state(self, input_signature): """Returns embedding vectors corresponding to input token IDs. Self._kernel_initializer = kernel_initializer Self._d_feature = d_feature # feature dimensionality Kernel_initializer: Function that creates (random) initial vectors for Vector to each id in `range(vocab_size)`.ĭ_feature: Dimensionality/depth of the output vectors.

Vocab_size: Size of the input vocabulary. """Returns an embedding layer with given vocabulary size and vector size. Kernel_initializer=init.RandomNormalInitializer(1.0)): """Trainable layer that maps discrete tokens/IDs to vectors.""" Tokenized_translation = tokenized_translation # Remove batch and EOS. Model, tokenized, temperature=0.0) # Higher temperature: more diverse results. Tokenized = tokenized # Add batch dimension. Tokenized = list((iter(), # Operates on streams. Sentence = 'It is nice to learn new things today!' Model.init_from_file('gs://trax-ml/models/translation/ende_', N_heads=8, n_encoder_layers=6, n_decoder_layers=6, # Pre-trained model config in gs://trax-ml/models/translation/ende_wmt32k.gin
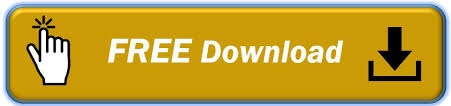